The Edge of Intelligence
Why today’s network cameras are so smart
- By Robert Muehlbauer
- May 01, 2013
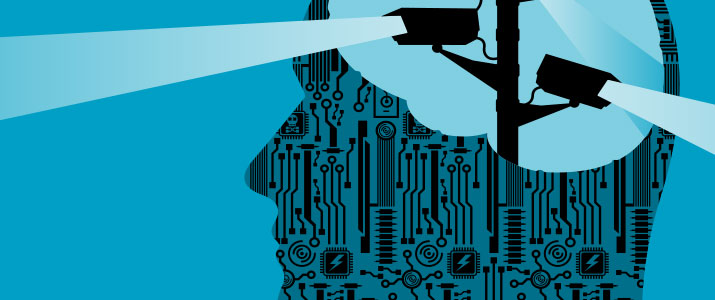
When the first network camera was introduced in 1996, its
functionality was pretty bare bones: digitize images and
send them across the network to a centralized video management
system. Its primary purpose was “Web attraction,”
where someone could log on to an Internet site and
view live situations, such as weather or traffic. But, as resolutions and frame
rates improved, it didn’t take long for manufacturers to realize the potential of
the camera’s built-in processor and redefine its role in security and surveillance
deployments.
By the mid-2000s, manufacturers introduced the first intelligent algorithm to
reside inside the camera—and since then, the industry has never looked back.
Processing Power: In-camera is in Demand
With processing power growth exceeding Moore’s Law in surveillance by more
than doubling every 18 months, intelligent analytics previously relegated to the
video server are now being performed in-camera. The cost-efficiencies of this shift
are significant:
- Lower bandwidth consumption—only pre-processed video is being sent across
the network rather than massive amounts of constantly streaming footage that
must be analyzed on the server side.
- Lower storage requirements—only content-rich video is being streamed to the
video storage array rather than every frame that was captured by the camera.
- Lower operating costs—in-camera processing is less expensive than monopolizing
CPU cycles on the server.
Motion Detection: Spotting an
Incident in Real Time
The first intelligent algorithm brought inside the camera
was motion detection. Pre-processing the video
in-camera meant the system could be set up so that no
video would be sent over the network unless there was
movement detected in the field of view. Not only did
this analytic cut down on bandwidth usage, it saved
on storage since non-event video wasn’t streamed to
the server for archiving. On the backend, users saved
on CPU cycles because the data center no longer had
to analyze all the footage for video motion before triggering
an alarmed event.
A common practice of business owners has been
to activate motion detection for after-hours surveillance,
curtailing video storage costs. For instance, a
store owner can stream and save all the video captured
during the 12 hours the store was open for business.
But once the store was closed for the day, the
motion-detection algorithm would only record video
if an event occurred. By strategically leveraging the
analytics, the store owner could conceivably cut video
storage costs in half.
Tamper Alarm: Protecting
the Field of View
The next algorithm to migrate to the camera was
an analytic to detect tampering attempts. If a camera
knocked away from its normal field of view, was
bumped out of focus or the lens was blocked—spray
painted or covered by an object—it automatically
sent an alert to the video management system to notify
security of the problem. This was a significant advantage
over analog systems, and is still an underused
intelligent analytic today.
The camera detects tampering by essentially
memorizing the pixel make-up of the scene it’s been
trained on, and sending an alarm when the pixels
change dramatically. Putting this ability in-camera
instead of waiting for the video management system
(VMS) to run periodic diagnostic cycles on edge devices
assures more immediate alerts about a camera’s
sub-performance. If the camera isn’t working or its
vision is blocked, the user will be notified.
Tampering alarm analytics have been effective in
schools where vandalism and playing pranks with
campus cameras are commonplace. Similar to motion
detection residing in-camera, the tampering
algorithm will automatically alert the VMS to the
problem, saving the process-intensive analytic from
having to run on the server. But equally important is
the immediacy of the alert, which would improve the
administration’s chances of catching the culprits in
the act and ensuring the camera can see once again.
Cross-line Detection:
Monitoring the Perimeter
Another analytic to take up residence in-camera was
cross-line detection—monitoring breaches in a perimeter.
The breach could occur in either direction—
somebody trying to break into a facility or trying to
exit the site without authorization, such as a prison
inmate attempting an escape. Cross-line detection also
could be used as a safety measure, for instance, on a
subway platform to ensure no one crossed onto the
track. In any case, the camera was now smart enough
to detect when a person, vehicle or object crossed the
invisible line and send an alarm to the video management
system to alert security staff to the event.
Oftentimes, outdoor perimeter installations face
challenges with connectivity and bandwidth. Running
cross-line detection in-camera provides the means
to trigger an immediate alarm, improving security’s
chances of apprehending the individual or protecting
people who enter restricted areas. Conversely, if security
has to wait for the back-end server to process
the cross-line detection, the window of opportunity
shrinks considerably.
Onboard Storage: SD and
microSD Memory Cards
Though the price of storage continues to drop, the
build out of server farms to support storage comes at
considerable expense, including the increased power
consumption to cool an ever-growing collection of
servers. In contrast, many of the newer network cameras
include slots for SD and microSD memory cards
that can store from 32 to 64 GB of video. As Moore’s
Law continues to drive down the cost per capacity and
physical size of storage devices, cameras and encoders
will soon support 2-plus TB of storage. In the realm
of video surveillance, this translates to about 55 days
of 1080p HDTV video running at 30 frames per second
using highly-efficient H.264 video compression.
Greater storage capacity, coupled with increased
processing power, opens a whole new range of possibilities
for the surveillance industry. For instance:
Self-contained solutions. Individual cameras and
video encoders can now be used as complete security
systems unto themselves. This provides a cost-effective
solution for environments with limited infrastructure
options. By embedding the video management system
in-camera, users could avoid the cost of external
storage and still remotely access the onboard video
directly. In outlying areas with limited bandwidth or
environments hostile to sensitive computer technology,
robust self-contained cameras could provide the
eyes, ears and analysis of the scene, as well as store
any forensic evidence. For instance, law enforcement
could deploy more standalone cameras in remote areas
of a city without increasing their existing centralized
storage capacity.
Redundancy for network outages. With greater
storage capacity in-camera, manufacturers will be
able to offer a level of fault tolerance for network outages
previously unattainable—even with applications
that require high resolution and real-time recording
rates. The camera now contains the intelligence to detect
an outage and automatically begin storing the images
in-camera until the connection is re-established
and video can once again be streamed over the network.
This is invaluable in deployments, such as casinos
where coverage is mandated by law and any loss
of video would incur stiff penalties.
Open Application Platforms:
Is There an App for That?
Leading IP cameras and video encoders now set aside a
certain amount of memory and CPU cycles dedicated
to running applications in-camera. Some manufactures
also support open application platforms that allow
third-party software developers to write custom analytics
that can run in-camera. This is creating an Appculture
in surveillance like the one pioneered by the
iPhone. A few popular third-party analytics include:
People and vehicle counting. These analytics provide
customer and operational insight in real time—
everything from the volume of foot and vehicle traffic
during specific hours to customer dwell time and suspicious
loitering. This helps store managers properly
staff their operations and manage customer interactions
with a higher level of service. Some universities
use object counting to help determine when a parking
garage is approaching capacity on game day.
License plate recognition. These analytics record
license plate images and compare them to images
stored on an internal SD memory card. If any plate
image matches a user-defined watch list, the camera
automatically sends an alert to the video management
system to inform security of any suspicious vehicles.
To work properly, however, this application requires a
camera with high resolution, good low-light capabilities
and a powerful processor.
Line management. This helps users facilitate the
flow of people in line and enhance customer service—
whether at a checkpoint or a checkout line.
The software links to an indicator line that tells an
individual where to stop and when to proceed to the
next available booth, desk or station. The analytic
tool can also be used by management to measure line
length and trigger an alert to open another checkout
bottleneck occurs.
These analytics were developed for the security
industry by some of the top surveillance developers
and VMS manufacturers. However, the next phase of
third-party applications will be the result of attracting
and encouraging developers from outside our industry
by opening their eyes to the opportunities in video
surveillance. Not only will this create more competition
to foster innovation, it will open up the market to
creative non-traditional surveillance uses.
As an industry, we should build partnerships with
academic institutions and support youth initiatives
like the FIRST Robotics Competition. This will empower
the next generation of software developers to
pursue careers in surveillance by providing an early
introduction to the true potential of in-camera intelligence.
Such initiatives are already starting to spark
some amazing and imaginative applications beyond
traditional security and surveillance—for everything
from analytics that help supervise processes on manufacturing
lines to algorithms designed to assist healthcare
providers in monitoring patient wellness.
The Future: Higher IQ Cameras
In recent years, the focus in network cameras has centered
on image resolution. Having progressed rapidly
from the grainy black-and-white images of the analog
CCTV days to the megapixel and HDTV full-color fidelity
of today’s IP systems, is it reasonable to project
50 and 100GB image processors within the next decade?
At some point, excellent image quality will exceed
what the eye can perceive and further resolution-based
advances will become a point of diminishing returns.
It seems more realistic to expect that increased
processing power will be directed towards expanding
intelligent analytics at the edge. What might some of
those more process-intensive applications be?
Facial recognition. Right now, facial recognition
algorithms require too much processing power to be
handled in-camera. But, with higher processing performance
and greater storage capacity being crammed
onto ever-smaller real estate, it’s reasonable to assume
that performance will soon reach a level where incamera
facial recognition analysis will be possible.
Metadata analysis. Extracting metadata directly
from the video in-camera appears to be the next big
breakthrough on the horizon. Being able to search
that metadata—for everything from the type of object
to the particular color of clothing—would be
significantly faster and far less expensive than searching
through the entire video archive. With sufficient
metadata you could narrow the search by designating
the type of object you’re looking for, whether it is a
person, vehicle, animal or something inanimate like a
rock. You could even search the video by the size of
the object and the direction the object was moving.
Smarter is Better
Today’s network cameras have matured from the
passive eyewitnesses of yesteryear into intelligent
computers that can evaluate what they see. The increased
processing performance has enabled network
cameras to take on a more vital role in analyzing,
managing and acting on events within their security
systems. By shifting more intelligent applications to
the edge, users not only save in bandwidth consumption,
storage and central processing costs, but can
process events and alerts in real time. This enables
faster searches for suspected activity and increases
the likelihood of resolving incidents more quickly—
turning electronic surveillance from a reactive to a
proactive industry.
This article originally appeared in the May 2013 issue of Security Today.